Published Aug 30, 2024
Why data integration is key for successful AI initiatives
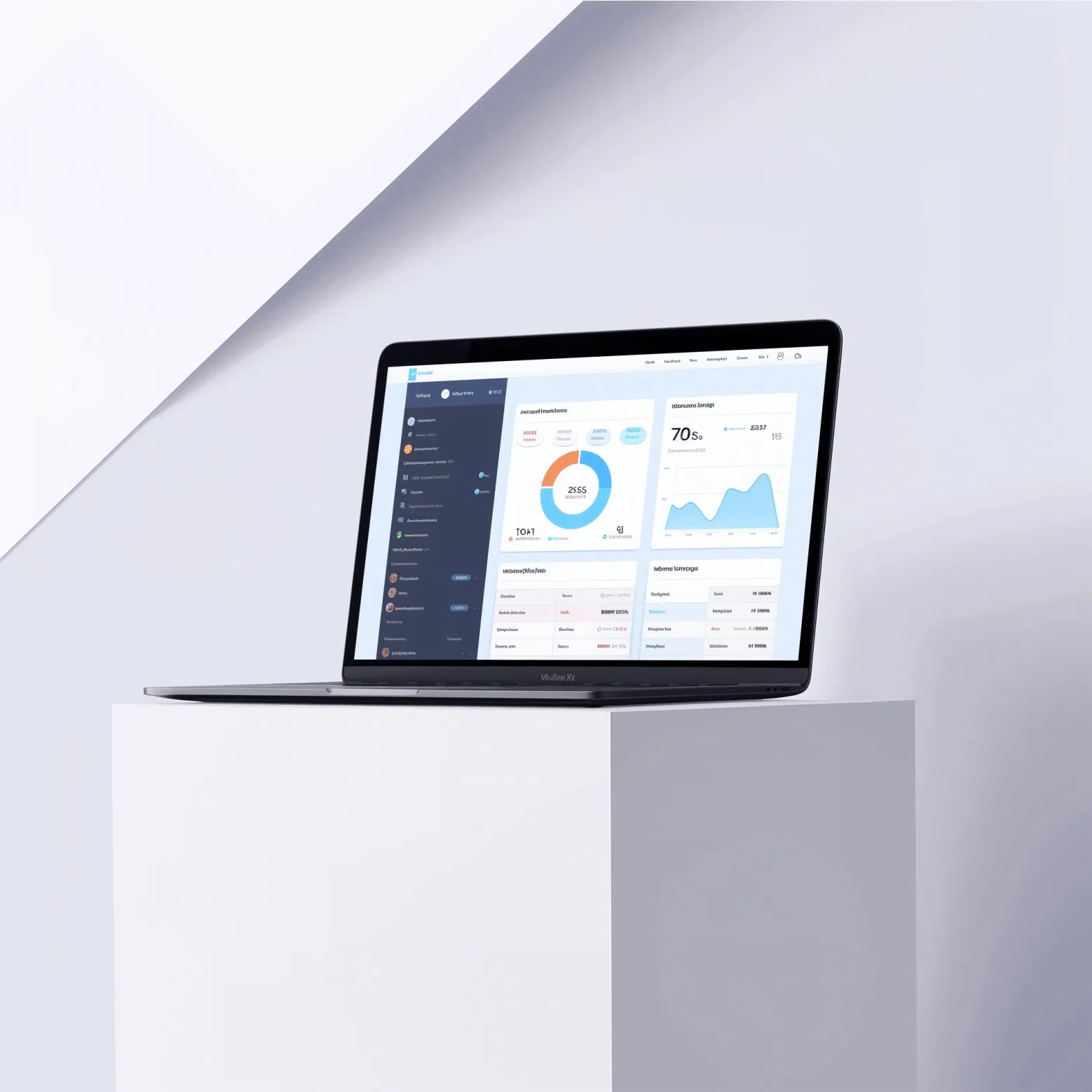
Integrating diverse data sources is critical for developing an AI strategy. As organizations work to build advanced AI systems, the ability to access, manage, and integrate data from various sources (such as cloud services, on-premise databases, IoT devices, and external APIs) becomes increasingly important.
This post explores how integrating diverse data sources is essential for an AI strategy, as it ensures that AI models have access to comprehensive, accurate, and up-to-date information, which enhances their ability to generate reliable and relevant insights. Data integration addresses limitations in large language models (LLMs) by filling data gaps, improving context, and enabling advanced applications like retrieval-augmented generation (RAG), ultimately maximizing the effectiveness and scalability of AI solutions.
The importance of data integration
Comprehensive data access
AI thrives on data, and the quality of this data directly influences the accuracy and reliability of AI models. To build robust AI systems, it’s essential to integrate data from a variety of sources. This integration ensures that a narrow or siloed view of the data landscape doesn’t limit AI models.
When AI systems can access diverse datasets, they develop a more holistic understanding of the context in which they operate. For example, in an ecommerce environment, integrating data from customer transactions, social media interactions, inventory systems, and market trends provides a comprehensive view that allows AI to make more accurate predictions about customer behavior, optimize supply chains, and personalize marketing efforts.
Data integration reduces the risk of hallucinations that can arise when models are trained on incomplete data. By drawing from a wide array of information, AI systems are better equipped to identify patterns, correlations, and trends that might otherwise go unnoticed. Comprehensive data integration allows AI to be more adaptive and responsive to real-world changes. As new data sources are added or existing ones evolve, the AI can incorporate this new information into its analysis, ensuring that its outputs remain relevant and up-to-date.
Enhanced data quality
Data quality is the cornerstone of successful AI initiatives. AI models are only as good as the data they are trained on, and poor-quality data can lead to inaccurate predictions, biased outcomes, and flawed decision-making. This is why ensuring high data quality is vital in any AI strategy.
When data is integrated from multiple sources, there’s a greater opportunity to implement comprehensive data governance strategies that enhance data quality. However, this process also introduces challenges, as data from different sources often varies in format, structure, and quality. Inconsistent data (such as duplicated records, missing values, or outdated information) can degrade the performance of AI models.
An iPaaS like Celigo is invaluable in addressing these challenges. An iPaaS facilitates data cleansing, validation, and enrichment at the integration stage, ensuring that only high-quality data feeds into AI models. By automating the removal of errors, standardizing data formats, and supplementing datasets with additional information, an iPaaS ensures that AI systems operate on a solid foundation of accurate and consistent data.
Streamlined AI workflows
Integrating diverse data sources simplifies the orchestration of AI workflows. By consolidating data into a unified platform, businesses can automate the entire AI pipeline—from data ingestion and processing to model training and deployment.
This automation reduces the need for extensive manual intervention, speeding up the development and deployment of AI applications and allowing organizations to respond quickly to changing business needs.
The role of data integration in RAG applications
Large language models (LLMs) often face limitations due to outdated context, data gaps, and a lack of access to domain-specific information, leading to inaccurate or unreliable outputs. Data integration addresses these issues by providing LLMs with up-to-date, comprehensive, and high-quality data, enhancing the accuracy and relevance of AI-driven applications like retrieval-augmented generation (RAG).
RAG is emerging as a pivotal technique for enhancing the capabilities of generative AI. RAG integrates data retrieval with text generation, allowing AI models to produce more accurate, contextually relevant, and informative responses. Implementing RAG effectively requires robust data integration and orchestration.
Why data integration is essential for RAG applications
Access to rich context
RAG applications enhance LLMs’ responses by retrieving relevant context from a variety of data sources. Integrating these diverse sources is essential because it ensures that AI can access the most relevant and comprehensive information available.
For example, by integrating internal knowledge bases, CRM systems, and real-time data feeds, AI can generate responses that are both accurate and contextually relevant, significantly improving the overall user experience.
Real-time data retrieval
RAG relies on the ability to retrieve the latest data in real-time. Real-time data integration is crucial because it ensures that the retrieval system can access and deliver up-to-date information to the LLM. This capability is especially important for applications like customer support, where timely and accurate responses are essential for maintaining customer satisfaction.
Improved accuracy and relevance
By leveraging integrated data, RAG applications can significantly improve the accuracy and relevance of their outputs. Data integration allows AI to cross-reference multiple data sources, verify information, and generate responses that are more precise and reliable.
For instance, integrating sales data, support tickets, and product documentation enables AI to provide detailed and accurate answers to customer inquiries, thereby enhancing the overall value and effectiveness of the AI application.
How an iPaaS facilitates data integration
Unified data access
An iPaaS like Celigo provides a unified platform for integrating a wide range of applications and data systems. This is achieved through prebuilt connectors and a robust API management framework, which allow disparate systems to communicate seamlessly.
By centralizing data access, an iPaaS eliminates data silos that can hinder an organization’s ability to get a complete view of its operations. With unified access, AI systems can leverage comprehensive datasets that encompass all relevant information across the enterprise. This holistic data environment not only enhances the accuracy of AI models but also improves their ability to deliver actionable insights, as they can now analyze data from a broader and more diverse set of sources.
Democratizes data integration
One of the key advantages of Celigo’s iPaaS is its low-code development environment, which democratizes the data integration process. Traditionally, integrating data across multiple systems required specialized IT resources with extensive coding knowledge. This often created bottlenecks, as businesses were dependent on a limited pool of technical experts to build and maintain integrations.
Celigo’s low-code platform empowers citizen integrators—business users with minimal coding experience—to take charge of data integration tasks. This democratization accelerates the development of AI applications, as more team members can contribute to the integration process. It also reduces the reliance on IT departments, enabling organizations to scale their AI efforts more rapidly and respond to evolving business needs with greater agility.
Access to real-time data
Celigo’s iPaaS supports real-time data integration, ensuring that AI models are constantly updated with the latest information. This real-time capability is particularly beneficial for applications that rely on up-to-the-minute data, such as predictive analytics, automated decision-making, and customer service platforms.
For example, in predictive analytics, real-time data integration allows AI models to analyze current trends and behaviors as they happen, leading to more accurate forecasts and recommendations. Similarly, in automated decision-making systems, the ability to process and act on real-time data is essential for making timely and relevant decisions.
Creating a successful AI strategy
Integrating diverse data sources is a foundational element of a successful AI strategy. By addressing the limitations of LLMs, enabling advanced applications like RAG, and ensuring the scalability, quality, and automation of AI workflows, data integration helps organizations maximize the value they derive from AI.
AI RESOURCES
Transform your AI strategy
Celigo’s advanced iPaaS opens the door of possibility, connecting all of your data, paving the way to deliver AI-powered solutions.